“AI isn’t just GenAI,” John Roese, Global CTO of Dell Technologies, said on a recent Tomorrow’s Tech Today webcast. “It’s a whole bunch of domains and it’s about moving work into machines.”
Are there capabilities allowing us to have machines not only develop, maintain and support, but also advertise and sell products?
IDC reports AI market to top $340 billion through 2021, even before GenAI became available. McKinsey expects AI to create $2.6 trillion to $4.4 trillion in global profits annually.
AI is not only GenAI
Traditional AI includes voice assistants like Siri or Alexa, recommendation engines on platforms like Netflix and Amazon, and Google’s search algorithm. These AIs are trained to follow specific rules and perform specific tasks, such as recognizing voice commands, recommending content based on user preferences, or ranking search results.
However, while they excel in their respective areas, these systems do not create new content or ideas independently. Instead, they operate within the parameters set by their training data and algorithms, making them highly efficient at solving particular problems but limited in creativity and adaptability beyond their predefined tasks.
Traditional AI systems primarily focus on analyzing existing data and making predictions based on patterns they recognize. They excel at tasks like classification, regression, and anomaly detection, providing insights into what the data reveals.
In contrast, generative AI takes this a step further by not only analyzing data but also creating new content or data that resembles its training material. While traditional AI can tell you what it sees in the data, generative AI uses that same data to produce entirely new outputs, such as text, images, or even music. In essence, traditional AI excels at pattern recognition, whereas generative AI specializes in pattern creation.
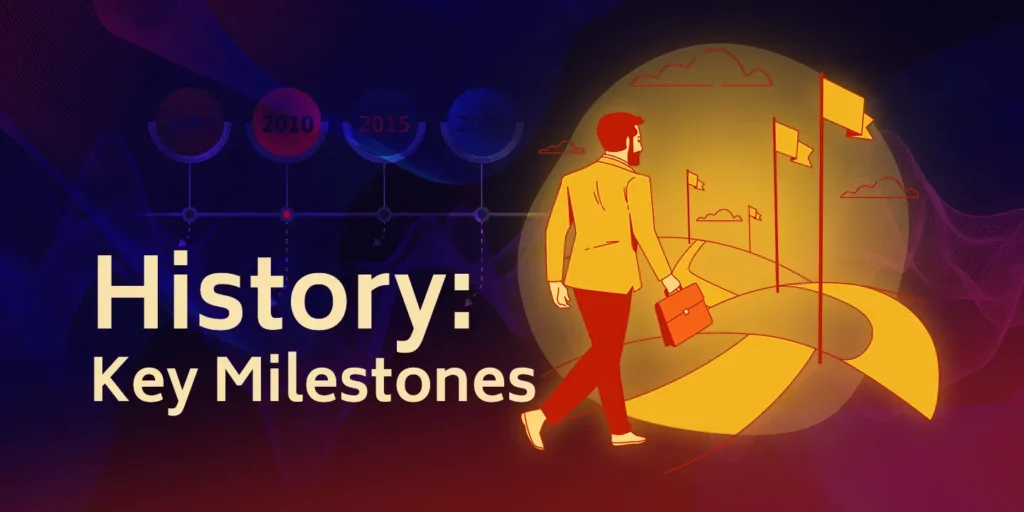
History: Key Milestones
Deep Blue and Chess (1997): IBM’s Deep Blue became the first computer to defeat reigning world chess champion Garry Kasparov, highlighting AI’s potential in complex problem-solving.
Deep Learning Revolution (2010s): A surge in deep learning, a subset of machine learning using artificial neural networks, transformed AI’s capabilities, particularly in image and speech recognition.
Rise of Generative Models (Mid-2010s): The introduction of generative models like GANs (Generative Adversarial Networks) enabled the creation of realistic images and artworks.
Language Processing Breakthroughs (2020): OpenAI’s GPT-3 marked a significant advancement in natural language processing, capable of tasks ranging from writing essays to coding.
AI for Lead Generation
Einstein by Salesforce: This AI-powered tool integrates with Salesforce’s CRM to analyze customer data, predicting buying behaviors and recommending promising leads. For example, a marketing agency used Einstein to prioritize leads, resulting in a 30% increase in sales productivity.
HubSpot’s AI Lead Scoring: HubSpot’s AI system ranks leads based on their potential value by analyzing historical data and user interactions. A technology startup reported a 25% increase in lead conversion rates after implementing this tool.
Drift’s AI Chatbots: Drift employs AI-powered chatbots to engage website visitors in real-time, qualifying leads by asking pre-programmed questions. A retail company reported a 40% increase in qualified leads due to the chatbot’s ability to engage customers 24/7.
LinkedIn Sales Navigator: Leveraging AI, Sales Navigator helps businesses find leads by analyzing user profiles and activities on LinkedIn. A financial services company noted a 20% increase in new client acquisitions attributed to the tool’s targeted suggestions based on customer preferences and search history.
MarketMuse: MarketMuse employs AI to analyze content and suggest engaging topics for target audiences. A content marketing agency saw a 50% increase in web traffic, boosting inbound leads significantly.
IBM Watson’s Personality Insights: This tool analyzes communication styles and personality traits. A business consultancy utilized it to tailor communication strategies, resulting in a 35% increase in engagement rates with leads.
AI in Paid Ads
Programmatic advertising has revolutionized digital marketing by automating real-time ad buying and selling. It started with real-time bidding (RTB) technology, which allows advertisers to bid for ad space in milliseconds. As the industry evolved, AI and machine learning were integrated to further enhance the efficiency of programmatic advertising. These technologies now play a key role in optimizing ad targeting, audience segmentation, and real-time adjustments, ensuring that ads reach the right audience at the most opportune moments, ultimately improving engagement and return on investment (ROI).
Techniques for maximizing ROI in programmatic ad buys using AI include:
- Predictive Creative Optimization (PCO): AI analyzes data to predict the best-performing ad creatives, ensuring relevance and engagement by adapting to trends and consumer behaviors.
- Cross-Channel Automated Optimization: Advanced algorithms optimize ad performance across platforms like social media and search engines, creating cohesive strategies by integrating insights from multiple channels.
- Fraud Detection and Prevention: AI identifies and prevents ad fraud by spotting patterns and anomalies, ensuring ad spend targets genuine audiences.
Examples of Successful AI-Driven Programmatic Campaigns:
- Kellogg’s: Through AI-powered programmatic ads with DoubleClick, Kellogg’s boosted ad visibility from 56% to over 70%, improving creative elements and placement for better performance.
- Local Now: Using AI tools with PubMatic, Local Now saw a 282% revenue increase and a substantial rise in ad requests, proving the impact of AI in driving growth and optimizing ad campaigns.
AI in Conversion Rate Optimization
Few or no digital marketing domains rely heavier on data than Conversion Rate Optimization. Proper audience targeting with subsequent content and UX adjustment secures visitors’ satisfaction which turns into customer success.
AI has transformed audience targeting and personalization in paid media by offering marketers tools that analyze and segment audiences with exceptional precision. Brands can now create personalized ads that align with individual consumer preferences and behaviors, maximizing engagement and ROI.
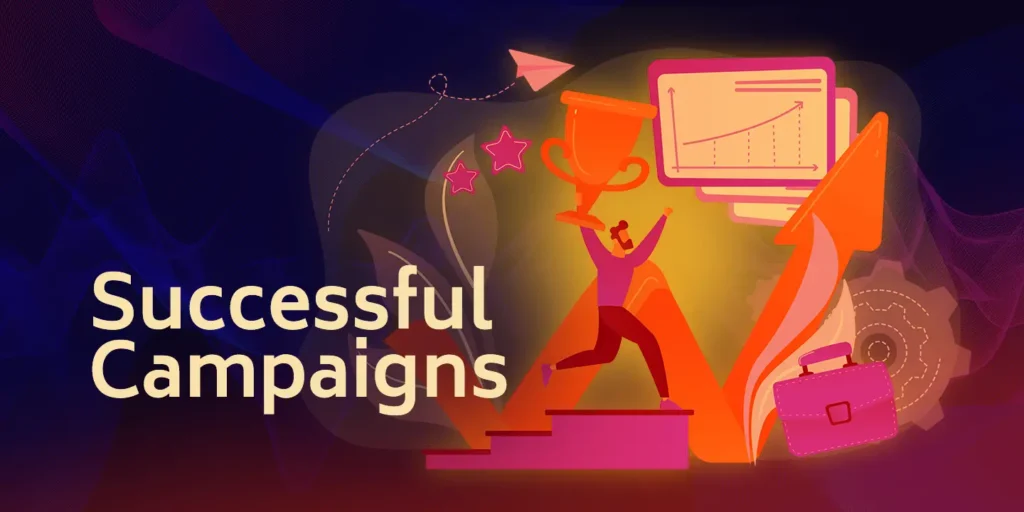
Success story: Booking.com
Booking.com leveraged AI to personalize the user experience on their platform. This resulted in a 65.16% increase in cart additions for returning visitors, a 73.72% boost in conversion rates, and a 16.15% increase in the average amount spent per transaction. This showcases the impact of AI-driven audience targeting and personalization on engagement and sales.
Success story: Procter & Gamble
Procter & Gamble (P&G) successfully leveraged AI-powered market segmentation to enhance ad targeting and drive impressive financial performance. By adopting a consumer-centric approach and utilizing data-driven insights, P&G was able to optimize its marketing efforts and target ads more effectively. This strategic use of AI contributed to substantial growth, with the company reporting sales reaching $84 billion and net earnings exceeding $10 billion.
The success of P&G highlights how AI can be a powerful tool for refining audience segmentation, enhancing ad relevance, and improving overall return on investment (ROI). By tailoring campaigns to consumer behaviors and preferences, P&G was able to achieve remarkable business results
Curious how Lerpal leverages AI and how we can fuel your business growth? Book a free demo consulting session with out CTO, CSO or submit an MVP request to prove your concept within days!
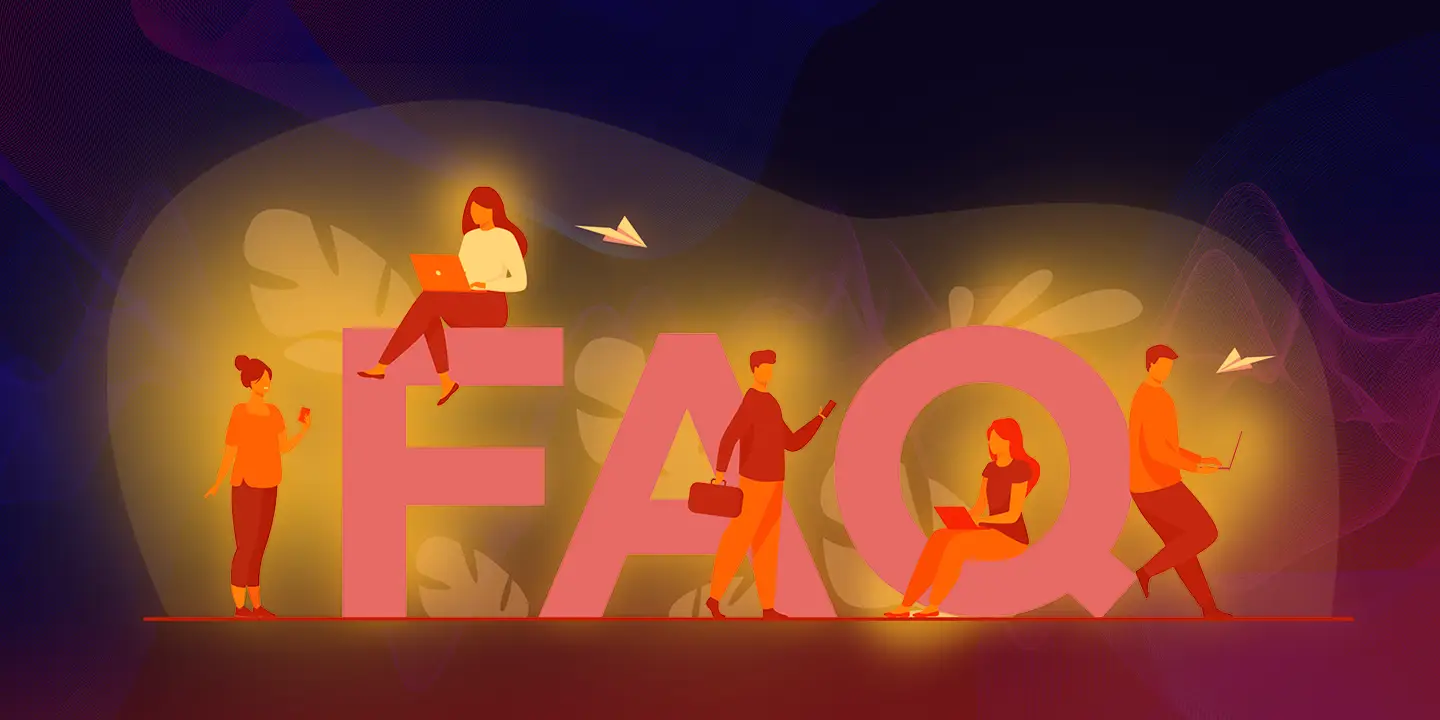
FAQ
Conversion Rate Optimization (CRO) is the process of enhancing a website’s performance to increase the percentage of users who take a desired action, such as making a purchase, adding items to a cart, or completing a form. Effective CRO strategies prioritize improving the customer experience, leading to higher conversion rates and better business outcomes.
Here are the five most significant branches of AI, each playing a unique role in the advancement of technology:
- Machine Learning (ML):
A subset of AI, ML enables machines to automatically learn from data and algorithms without needing explicit programming. By leveraging patterns in data, ML helps machines make decisions autonomously. - Deep Learning (DL):
A specialized form of ML, DL utilizes artificial neural networks (ANNs) inspired by the human brain. These networks allow machines to extract complex patterns and representations from large datasets, leading to advanced performance. DL is behind technologies like virtual assistants such as Amazon Alexa and Google Home. - Natural Language Processing (NLP):
NLP enables computers to understand and interpret human language, both written and spoken. It is commonly used in digital assistants, chatbots, and sentiment analysis tools that assess the emotions expressed in text about a product or service. - Robotics:
Robotics combines AI with machine design to create machines capable of performing tasks autonomously. This field often involves integrating other AI technologies, such as ML and NLP, to allow robots to function in industries like healthcare, retail, and manufacturing. - Fuzzy Logic:
Fuzzy logic helps AI systems handle uncertainty and imprecise information, enabling them to make decisions in conditions that are not strictly true or false. It’s commonly used in systems like automatic braking in cars, adjusting based on variable conditions.
Lead generation with data involves using web scraping to extract valuable leads from various online sources. It automates the process of collecting information such as contact details, preferences, and behaviors, providing businesses with a targeted and efficient approach to identifying potential customers.
Web scraping can target a diverse range of sources, including business directories, social media platforms (Twitter, LinkedIn, Facebook), job boards, industry-specific websites, and more. This ensures a comprehensive extraction of leads from various channels.
Lead data enhances sales and marketing efforts by allowing businesses to create targeted marketing campaigns, personalize communication, improve conversion rates, and make data-driven decisions that align with the preferences and behaviors of potential customers.
Data parsing involves converting data from one format into another, typically for structuring and organizing it in a more comprehensible and usable way. It’s a common process for transforming unstructured or complex data into readable, structured formats. For example, data parsing might be used to convert an unreadable HTML file into plain text, making it easier for users to understand.
This process is applied across various industries, including finance, education, sports, and retail, to extract key information efficiently. It saves time and effort by automating data extraction, reducing the need for manual processing to obtain accurate and useful data.
Digital marketing refers to the use of online platforms, such as websites, mobile apps, social media, search engines, and other digital channels, to promote and sell products or services. While it shares foundational principles with traditional marketing—such as understanding consumer behavior and targeting specific audiences—it offers unique strategies and tools for reaching customers in the digital age.
Digital marketing gained prominence in the 1990s with the rise of the internet and has since become integral to most companies’ marketing strategies. Many businesses use a combination of both traditional and digital marketing methods to maximize their reach and effectiveness. However, digital marketing presents its own set of challenges, such as staying current with technological advancements and navigating data privacy regulations.
Conversion rate optimization (CRO) focuses on improving the percentage of users who complete a desired action on a website, such as making a purchase, signing up for a newsletter, or filling out a form. By enhancing user experience and reducing friction points, CRO aims to turn more visitors into customers or leads.
Successful CRO strategies involve analyzing user behavior, identifying barriers to conversion, and implementing changes to improve usability, navigation, and content. This can involve A/B testing different versions of webpages, optimizing load times, simplifying checkout processes, or personalizing content to better meet user needs.